How FDA Regulates Artificial Intelligence in Medical Products
As technology evolves, oversight will need to keep pace
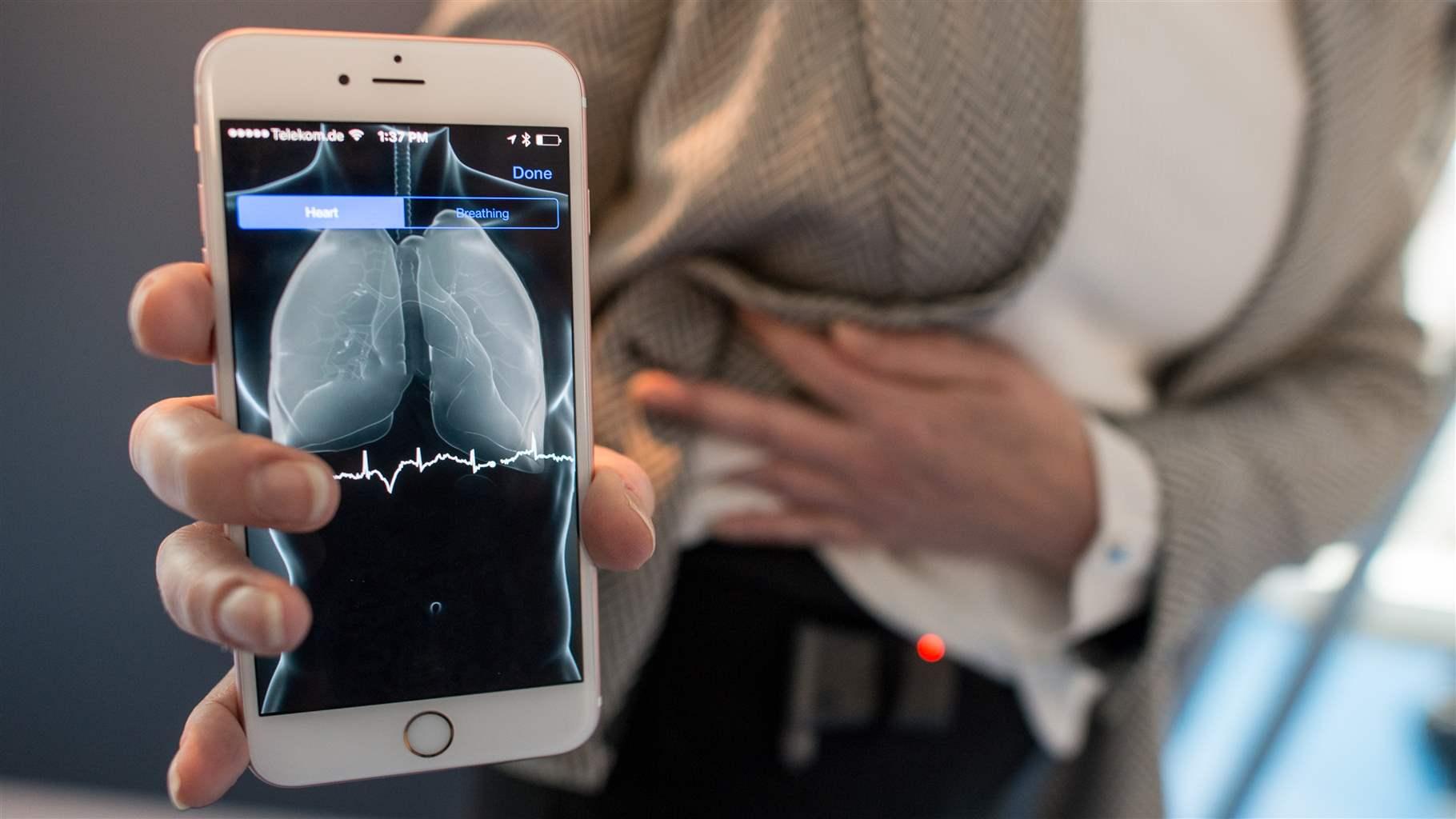
Overview
Health care organizations are using artificial intelligence (AI)—which the U.S. Food and Drug Administration defines as “the science and engineering of making intelligent machines”—for a growing range of clinical, administrative, and research purposes. This AI software can, for example, help health care providers diagnose diseases, monitor patients’ health, or assist with rote functions such as scheduling patients.
Although AI offers unique opportunities to improve health care and patient outcomes, it also comes with potential challenges. AI-enabled products, for example, have sometimes resulted in inaccurate, even potentially harmful, recommendations for treatment.1 These errors can be caused by unanticipated sources of bias in the information used to build or train the AI, inappropriate weight given to certain data points analyzed by the tool, and other flaws.
The regulatory framework governing these tools is complex. FDA regulates some—but not all—AI-enabled products used in health care, and the agency plays an important role in ensuring the safety and effectiveness of those products under its jurisdiction. The agency is currently considering how to adapt its review process for AI-enabled medical devices that have the ability to evolve rapidly in response to new data, sometimes in ways that are difficult to foresee.2
This brief describes current and potential uses of AI in health care settings and the challenges these technologies pose, outlines how and under what circumstances they are regulated by FDA, and highlights key questions that will need to be addressed to ensure that the benefits of these devices outweigh their risks. It will take a collective effort by FDA, Congress, technology developers, and the health care industry to ensure the safety and effectiveness of AI-enabled technology.
What is AI and how is it used in health care?
AI refers to the ability of a machine to perform a task that mimics human behavior, including problem-solving and learning.3 It can be used for a range of purposes, including automating tasks, identifying patterns in data, and synthesizing multiple sources of information. In health care, AI technologies are already used in fields that rely on image analysis, such as radiology and ophthalmology, and in products that process and analyze data from wearable sensors to detect diseases or infer the onset of other health conditions.4
AI programs can also predict patient outcomes based on data collected from electronic health records, such as determining which patients may be at higher risk for disease or estimating who should receive increased monitoring. One such model identifies patients in the emergency room who may be at increased risk for developing sepsis based on factors such as vital signs and test results from electronic health records.5 Another hospital system has developed a model that aims to better predict which discharged patients are likely to be readmitted following their release compared with other risk-assessment tools.6 Other health care systems will likely follow suit in developing their own models as the technology becomes more accessible and well-established, and as federal regulations implement efforts to facilitate data exchange between electronic health record systems and mobile applications, a process known as interoperability.7
Finally, AI can also play a role in research, including pharmaceutical development, combing through large sets of clinical data to improve a drug’s design, predict its efficacy, and discover novel ways to treat diseases.8 The COVID-19 pandemic might help drive advances in AI in the clinical context, as hospitals and researchers have deployed it to support research, predict patient outcomes, and diagnose the disease.9 Some examples of AI products developed for use against COVID-19:10
-
COViage, a software prediction system, assesses whether hospitalized COVID-19 patients are at high risk of needing intubation.11
-
CLEWICU System, prediction software that identifies which ICU COVID-19 patients are at risk for respiratory failure or low blood pressure.12
-
Mount Sinai Health System developed an AI model that analyzes computed tomography (CT) scans of the chest and patient data to rapidly detect COVID-19.13
-
Researchers at the University of Minnesota, along with Epic Systems and M Health Fairview, developed an AI tool that can evaluate chest X-rays to diagnose possible cases of COVID-19.14
How are AI products developed?
AI can be developed using a variety of techniques. In traditional, or rules-based, approaches, an AI program will follow human-prescribed instructions for how to process data and make decisions, such as being programmed to alert a physician each time a patient with high blood pressure should be prescribed medication.15 Rules-based approaches are usually grounded in established best practices, such as clinical practice guidelines or literature.16 On the other hand, machine learning (ML) algorithms—also referred to as a data-based approach—“learn” from numerous examples in a dataset without being explicitly programmed to reach a particular answer or conclusion.17 ML algorithms can learn to decipher patterns in patient data at scales larger than a human can analyze while also potentially uncovering previously unrecognized correlations.18 Algorithms may also work at a faster pace than a human. These capabilities could be especially useful in health care settings, which can provide continuous streams of data from sources, including patient medical records and clinical studies.19
Most ML-driven applications use a supervised approach in which the data used to train and validate the algorithm is labeled in advance by humans; for example, a collection of chest X-rays taken of people who have lung cancer and those who do not, with the two groups identified for the AI software. The algorithm examines all examples within the training dataset to “learn” which features of a chest X-ray are most closely correlated with the diagnosis of lung cancer and uses that analysis to predict new cases. Developers then test the algorithm to see how generalizable it is; that is, how well it performs on a new dataset, in this case, a new set of chest X-rays. Further validation is required by the end user, such as the health care practice, to ensure that the algorithm is accurate in real-world settings. Unsupervised learning is also possible, in which an algorithm does not receive labeled data and instead infers underlying patterns within a dataset.20
Challenges and risks with AI-enabled products
Like any digital health tool, AI models can be flawed, presenting risks to patient safety. These issues can stem from a variety of factors, including problems with the data used to develop the algorithm, the choices that developers make in building and training the model, and how the AI-enabled program is eventually deployed.
AI programs should be built and trained appropriately
AI algorithms need to be trained on large, diverse datasets to be generalizable across a variety of populations and to ensure that they are not biased in a way that affects their accuracy and reliability. These challenges can resemble those for other health care products. For example, if a drug is tested in a clinical trial population that is not sufficiently representative of the actual populations it will be used in, it will not work as well when implemented in real-world clinical settings. In AI, similarly, any model must be evaluated carefully to ensure that its performance can be applied across a diverse set of patients and settings.
However, such datasets are often difficult and expensive to assemble because of the fragmented U.S. health care system, characterized by multiple payers and unconnected health record systems. These factors can increase the propensity for error due to datasets that are incomplete or inappropriately merged from multiple sources.21 A 2020 analysis of data used to train image-based diagnostic AI systems found that approximately 70% of the studies that were included used data from three states, and that 34 states were not represented at all. Algorithms developed without considering geographic diversity, including variables such as disease prevalence and socioeconomic differences, may not perform as well as they should across a varied array of real-world settings.22
The data collection challenges and the inequalities embedded within the health care system contribute to bias in AI programs that can affect product safety and effectiveness and reinforce the disparities that have led to improper or insufficient treatment for many populations, particularly minority groups.23 For example, cardiovascular disease risks in populations of races and ethnicities that are not White have been both overestimated and underestimated by algorithms trained with data from the Framingham Heart Study, which mostly involved White patients.24 Similarly, if an algorithm developed to help detect melanoma is trained heavily on images of patients with lighter skin tones, it may not perform as well when analyzing lesions on people of color, who already present with more advanced skin disease and face lower survival rates than White patients.25
Bias can also occur when an algorithm developed in one setting, such as a large academic medical center, is applied in another, such as a small rural hospital with fewer resources. If not adapted and validated for its new context, an AI program may recommend treatments that are not available or appropriate in a facility with less access to specialists and cutting-edge technology.26
Moreover, assembling sufficiently large patient datasets for AI-enabled programs can raise complex questions about data privacy and the ownership of personal health data. Protections to ensure that sensitive patient data remains anonymous are vital.27 Some health systems are sharing their patients’ data with technology companies and digital startups to develop their AI-based programs, sometimes without those patients’ knowledge. There is ongoing debate over whether patients should consent to having their data shared, and whether they should share in the profits from products that outside entities develop using their data.28 However, anonymizing patient data can pose its own challenges, as it can sometimes undermine efforts to ensure the representativeness of large datasets; if patient demographics are unknown to AI developers, then they may not be able to detect bias in the data.
Ensuring safe and effective use of AI-enabled products
Once an AI-enabled program has been developed, it must be used in a way that ensures that it consistently performs as expected. This can be a complex undertaking, depending on the purpose of the AI model and how it is updated.
ML algorithms, for example, fall along a spectrum from “locked” to “adaptive” (also referred to as “continuous learning”). In a locked algorithm, the same input will always produce the same result unless the developer updates the program. In contrast, an adaptive algorithm has the potential to update itself based on new data, meaning that the same input could generate different decisions and recommendations over time.29 Either type of algorithm presents its own challenges.
Locked algorithms can degrade as new treatments and clinical practices arise or as populations alter over time. These inevitable changes may make the real-world data entered into the AI program vastly different from its training data, leading the software to yield less accurate results. An adaptive algorithm could present an advantage in such situations, because it may learn to calibrate its recommendations in response to new data, potentially becoming more accurate than a locked model. However, allowing an adaptive algorithm to learn and adapt on its own also presents risks, including that it may infer patterns from biased practices or underperform in small subgroups of patients.30
AI-enabled programs can also pose risks if they are not deployed appropriately and monitored carefully. One study found that a widely used algorithm disproportionately recommended White patients for high-risk care management programs, which provide intensive—and often expensive—services to people with complex health needs. Several health systems relied on the algorithm to identify patients who were most likely to benefit. However, the algorithm used higher health care costs as a proxy for medical need. Because Black patients are less likely to have access to care, even if they are insured, their health care costs tend to be lower. As a result, the algorithm systematically underestimated their health needs and excluded them from high-risk care programs.31
Other challenges relate to the explainability of the output—that is, how easy it is to explain to the end user how a program produced a certain result—and the lack of transparency around how an AI-enabled program was developed. Some AI programs, for example, are referred to as “black-box” models because the algorithms are derived from large datasets using complex techniques and reflect underlying patterns that may be too convoluted for a person, including the initial programmer, to understand. AI companies may also choose to keep their algorithms confidential, as proprietary information.32 Moreover, companies do not always publicly report detailed information on the datasets they use to develop or validate algorithms, limiting the ability of health care providers to evaluate how well the AI will perform for their patients. For example, a report examining companies’ public summaries about their FDA-approved AI tools found that, of the 10 products approved for breast imaging, only one included a breakdown of the racial demographics in the dataset used to validate the algorithm. Breast cancer is significantly more likely to be fatal in Black women, who may be diagnosed at later stages of the disease and who experience greater barriers to care. Some or all of the AI devices in question may have been trained and validated on diverse patient populations, but the lack of public disclosure means that health care providers and patients might not have all the information they need to make informed decisions about the use of these products.33
In addition, patients are often not aware when an AI program has influenced the course of their care; these tools could, for example, be part of the reason a patient does not receive a certain treatment or is recommended for a potentially unnecessary procedure.34 Although there are many aspects of health care that a patient may not fully understand, in a recent patient engagement meeting hosted by FDA, some committee members—including patient advocates—expressed a desire to be notified when an AI product is part of their care. This desire included knowing if the data the model was trained on was representative of their particular demographics, or if it had been modified in some way that changed its intended use.35
Given the complexity of these products and the challenge of deploying them, health systems may need to recruit or train staff members with the technical skills to evaluate these models, understand their limitations, and implement them effectively. A provider’s trust in—and ability to correctly and appropriately use—an AI tool is fundamental to its safety and effectiveness, and these human factors may vary significantly across institutions and even individuals.36 If providers do not understand how and why an algorithm arrived at a particular decision or result, they may struggle to interpret the result or apply it to a patient.
Software developers, health care providers, policymakers, and patients all have a role to play in addressing these various challenges. Regulatory agencies also may need to adapt their current oversight processes to keep pace with the rapid shifts underway in this field.
How and under what circumstances does FDA regulate AI products?
FDA is tasked with ensuring the safety and effectiveness of many AI-driven medical products. The agency largely regulates software based on its intended use and the level of risk to patients if it is inaccurate. If the software is intended to treat, diagnose, cure, mitigate, or prevent disease or other conditions, FDA considers it a medical device.37 Most products considered medical devices and that rely on AI/ML are categorized as Software as a Medical Device (SaMD).38 Examples of SaMD include software that helps detect and diagnose a stroke by analyzing MRI images, or computer-aided detection (CAD) software that processes images to aid in detecting breast cancer.39 Some consumer-facing products—such as certain applications that run on a smartphone—may also be classified as SaMD.40 By contrast, FDA refers to a computer program that is integral to the hardware of a medical device—such as one that controls an X-ray panel—as Software in a Medical Device.41 These products can also incorporate AI technologies.
Examples of FDA Cleared or Approved AI-Enabled Products
![]() |
IDx-DR: Detects diabetic retinopathyThis software analyzes images of the eye to determine whether the patient should be referred to an eye professional because the images portray more than mild diabetic retinopathy or the patient should be rescreened in a year because the images were negative for more than mild diabetic retinopathy.42 |
![]() |
OsteoDetect: Detects and diagnoses wrist fracturesThis software analyzes X-rays for signs of distal radius fracture and marks the location of the fracture to aid in detection and diagnosis.43 |
![]() |
ContaCT: Detects a possible stroke and notifies a specialistThis software analyzes CT images of the brain for indicators usually associated with a stroke, and immediately texts a specialist if a suspected large vessel blockage is identified, potentially involving the specialist sooner than the usual standard of care.44 |
![]() |
Guardian Connect System: Continuous glucose monitoring systemThis product monitors glucose levels in the tissues of a diabetic patient, using a sensor inserted under the skin, either on an arm or on the abdomen. A transmitter processes and sends this information wirelessly to an application installed on a mobile device. Patients can use the program to monitor whether their glucose levels are too low or high.45 |
![]() |
Embrace2: Wearable seizure monitoring deviceThis product monitors physiological signals through a device worn on the wrist. If the technology senses activity that may indicate a seizure, it will send a command to a paired wireless device programmed to alert the patient’s designated caregiver. The system will also record and store data from its sensors for future review by a health care professional.46 |
![]() |
FibriCheck: Mobile application to detect atrial fibrillationThis application uses either a smartphone camera or sensors in a smartwatch to analyze and record heart rhythms. This information, along with symptoms the patient is prompted to enter, are aggregated into a report along with next steps for the patient, if necessary.47 |
As with any medical device, AI-enabled software is subject to FDA review based on its risk classification. Class I devices—such as software that solely displays readings from a continuous glucose monitor— pose the lowest risk. Class II devices are considered to be moderate to high risk, and may include AI software tools that analyze medical images such as mammograms and flag suspicious findings for a radiologist to review.48 Most Class II devices undergo what is known as a 510(k) review (named for the relevant section of the Federal Food, Drug, and Cosmetic Act), in which a manufacturer demonstrates that its device is “substantially equivalent” to an existing device on the market with the same intended use and technological characteristics.49 One study found that the majority of FDA-reviewed AI-based devices on the market have come through FDA’s 510(k) pathway. However, the authors note that they relied on publicly available information, and because the agency does not require companies to categorize their devices as AI/ML-based in public documents, it is difficult to know the true number.50
Alternatively, certain Class I and Class II device manufacturers may submit a De Novo request to FDA, which can be used for devices that are novel but whose safety and underlying technology are well understood, and which are therefore considered to be lower risk.51 Several AI-driven devices currently on the market—such as IDx-DR, OsteoDetect, and ContaCT (see the text box, “Examples of FDA Cleared or Approved AI-Enabled Products”)—are Class II devices that were reviewed through the De Novo pathway.52
Class III devices pose the highest risk. They include products that are life-supporting, life-sustaining, or substantially important in preventing impairment of human health. These devices must undergo the full premarket approval process, and developers must submit clinical evidence that the benefits of the product outweigh the risks.53 The continuous glucose monitoring system, Guardian Connect system, was approved through a premarket approval.54
Once a device is on the market, FDA takes a risk-based approach to determine whether it will require premarket review of any changes the developer makes. In general, each time a manufacturer significantly updates the software or makes other changes that would substantially affect the device’s performance, the device may be subject to additional review by FDA, although the process for this evaluation differs depending on the device’s risk classification and the nature of the change.
Exemptions From FDA Review
Congress excluded certain health-related software from the definition of a medical device in the 21st Century Cures Act of 2016.55 Exemptions in the law include software that:
-
Is intended for administrative support of a health care facility.
-
Example: software for scheduling, practice and inventory management, or to process and maintain financial records.
-
-
Is intended for maintaining or encouraging a healthy lifestyle and is unrelated to the diagnosis, cure, mitigation, prevention, or treatment of a disease or condition.
-
Example: mobile applications that actively monitor exercise, provide daily motivational tips to reduce stress, or offer tools to promote or encourage healthy eating.
-
-
Is intended to serve as electronic patient records, including patient-provided information, and its function is not intended to interpret or analyze patient records (including imaging data) for the purpose of diagnosis, cure, mitigation, prevention, or treatment.
-
Examples: mobile applications that allow patients with a certain medical condition to record measurements or other events to share with their health care provider as part of a disease management plan, or that allow health care providers to access their patient’s personal health record hosted on a web-based or other platform.
-
-
Is intended for transferring, storing, converting formats, or displaying data or results.
-
Example: software functions that display medical device data without modifying the data.
-
-
Is not intended to acquire, process, or analyze data from scanning or diagnostic devices such as MRIs or in vitro clinical tests, AND is used for the purpose of:
-
Displaying, analyzing, or printing medical data, such as patient information or peer-reviewed clinical studies.
-
Supporting or providing medical recommendations to a health care professional, on the condition that the software allows the provider to independently review how those recommendations were made.
-
-
Example: certain clinical decision support software.
Source: 21st Century Cures Act of 2016, Food and Drug Administration
Clinical decision support (CDS) software is a broad term that FDA defines as technologies that provide health care providers and patients with “knowledge and person-specific information, intelligently filtered or presented at appropriate times to enhance health and health care.”56 Studies have shown that CDS software can improve patient care.57 These products can have device and nondevice applications. To be exempt from the definition of device, and not regulated by the FDA, CDS software must meet criteria that Congress set in the 21st Century Cures Act of 2016. (See the text box, “Exemptions From FDA Review.”)
Crucially, the CDS software must support or provide recommendations to health care providers as they make clinical decisions, but the software cannot be intended toreplace a provider’s independent judgment. That is, the software can inform decisions, but it cannot be intended as the driving factor behind them. Otherwise, the software must be regulated as a medical device by the agency. The distinction between informing and driving a decision can be difficult to assess and has proved challenging for FDA to describe as it attempts to implement the law. The agency released draft guidance in 2017 on how it would interpret those provisions with respect to CDS software. In response to feedback from product developers, which raised concerns that the agency was interpreting its authority too broadly, FDA officials revised and re-released the draft guidance in 2019. However, the 2019 guidance—in which FDA attempted to harmonize its interpretation of the 21st Century Cures Act with existing international criteria for software—has also drawn concerns from some health care provider organizations. They argue that the guidance may exclude too many types of software from review and that FDA needs to clarify how the agency would apply it to specific products.58
This is particularly the case for CDS products—including those that rely on AI—developed and used by health care providers. Some health systems may be developing or piloting AI-driven CDS software for use within their own facility that might technically meet the definition of a medical device. The distinction between the practice of medicine—which FDA does not regulate—and a device is unclear in circumstances in which a software program is developed and implemented within a single health care system and is not sold to an outside party. The agency has not publicly stated its position on this issue; however, current regulations do exempt licensed practitioners who manufacture or alter devices solely for use in their practice from product registration requirements.59
Hospital accrediting bodies (such as the Joint Commission), standards-setting organizations (such as the Association for the Advancement of Medical Instrumentation), and government actors may need to fill this gap in oversight to ensure patient safety as these tools are more widely adopted.60 For example, the Federal Trade Commission (FTC), which is responsible for protecting consumers and promoting fair market competition, published guidance in April 2020 for organizations using AI-enabled algorithms. Because algorithms that automate decision-making have the potential to produce negative or adverse outcomes for consumers, the guidance emphasizes the importance of using tools that are transparent, fair, robust, and explainable to the end consumer.61 One year later, the FTC announced that it may take action against those organizations whose algorithms may be biased or inaccurate.62
Emerging FDA proposals for SaMD regulation
FDA officials have acknowledged that the rapid pace of innovation in the digital health field poses a significant challenge for the agency. They say new regulatory frameworks will be essential to allow the agency to ensure the safety and effectiveness of the devices on the market without unnecessarily slowing progress.63
In 2019, the agency began piloting an oversight framework called the Software Precertification Program, which, if fully implemented, would be a significant departure from its normal review process. Rather than reviewing devices individually, FDA would first evaluate the developer. If the organization meets certain qualifications and demonstrates it has rigorous processes to develop safe, effective devices, it would be able to undergo a significantly streamlined review process and make changes or even introduce products without going through premarket review. Nine companies participated in this pilot program. The lessons learned may help inform the development of a future regulatory model for software-based medical devices.64 However, some members of Congress have questioned FDA’s statutory authority to establish this program.65 Legislation may be required before FDA can fully implement it, and its appeal to software developers is not yet clear.
The agency has also proposed a regulatory framework targeted to SaMD products that rely on an adaptive learning approach. Thus far, FDA has only cleared or approved AI devices that rely on a “locked” algorithm, which does not change over time unless it is updated by the developer. Adaptive algorithms, by contrast, have the potential to incorporate new data and “learn” in real time, meaning that the level of risk or performance of the product may also change rapidly. Given the speed and sometimes unpredictable nature of these changes, it can be difficult to determine when the SaMD’s algorithm may require additional review by the agency to ensure that it is still safe and effective for its intended use.
In a 2019 white paper, FDA outlined a potential approach to addressing this question of adaptive learning. It is based on four general principles:66
-
Clear expectations on quality systems and good machine learning practices. As with any device manufacturer, FDA expects SaMD developers to have an established system to ensure that their device meets the relevant quality standards and conforms to regulations. In addition, a developer would need to implement established best practices for developing an algorithm, known as Good Machine Learning Practices (GMLP). This set of standards is still evolving, and may eventually need to be included as an amendment to current Good Manufacturing Practice requirements for devices.67 FDA has recently stated it needs industry and stakeholder input to address outstanding questions on what these good practices look like for algorithm design, training, and testing.68
-
Premarket assessment of SaMD products that require it. Under this framework, developers would have the option to submit a plan for future modifications, called a predetermined change control plan, as part of the initial premarket review of an SaMD that relies on AI/ML. This plan would include the types of anticipated modifications that may occur and the approach the developer would use to implement those changes and reduce the associated risks.
-
Routine monitoring of SaMD products by manufacturers to determine when an algorithm change requires FDA review. Under the current regulatory framework, many changes to an SaMD product would likely require the developer to file a new premarket submission. In the proposed approach, if modifications are made within the bounds of the predetermined change control plan, developers would need only to document those changes. If the changes are beyond the scope of the change control plan but do not lead to a new intended use of the device (for example, the developer makes the SaMD compatible with other sources of data, or incorporates a different type of data), then FDA may perform a review of the change control plan alone and approve a new version. However, if the modifications lead to a new intended use (for example, by expanding the target patient population from adults to children), then FDA would likely need to conduct an additional premarket review.
-
Transparency and real-world performance monitoring. As part of this approach, FDA would expect a commitment from developers to adhere to certain principles of transparency and engage in ongoing performance monitoring. As such, developers would be expected to provide periodic reporting to the agency on implemented updates and performance metrics, among other requirements.
The proposed framework would be a significant shift in how FDA currently regulates devices, and—as with the precertification program—the agency has acknowledged that certain aspects of the framework may require congressional approval to implement.69 Even if permission is granted, there are outstanding questions about how this framework would be implemented in practice and applied to specific devices. FDA is currently working on a series of follow-up documents that will provide further details on its proposed approach.70
Most recently, the agency published the “Artificial Intelligence/Machine Learning (AI/ML)-Based Software as a Medical Device (SaMD) Action Plan,” outlining its intended next steps. These include updating its proposed framework and issuing draft guidance on the predetermined change control plan, encouraging harmonization among technology developers on the development of GMLP, and holding a public workshop on medical device labeling to support transparency to end users. In addition, the agency will support efforts to develop methods for the evaluation and improvement of ML algorithms, including how to identify and eliminate bias, and to work with stakeholders to advance real-world performance monitoring pilots.71
Remaining questions and oversight gaps
Especially as the use of AI products in health care proliferates, FDA and other stakeholders will need to develop clear guidelines on the clinical evidence necessary to demonstrate the safety and effectiveness of such products and the extent to which product labels need to specify limitations on their performance and generalizability. As part of this effort, the agency could consider requiring developers to provide public information about the data used to validate and test AI devices so that end users can better understand their benefits and risks.
FDA’s recent SaMD Action Plan is a good step forward, but the agency will still need to clarify other key issues, including:
-
When a modification to SaMD or an adaptive ML device requires premarket review. The draft guidance on the predetermined change control plan could be a critical part of this policy.
-
Whether and how the Software Precertification Program can be extended beyond the pilot phase.
-
The distinction between software regulated by FDA and exempt software, which will turn heavily on the difference between informing clinical decisions and driving them.
-
How GMLP, when they are developed, will intersect with the current quality system regulations that apply to all devices.
-
How software updates and potential impacts on performance will be communicated to end users.
In addition, because there are products otherwise excluded from the definition of a medical device, another oversight body may need to play a role in ensuring patient safety, particularly for AI-enabled software not subject to FDA’s authority. Further, for AI products used in the drug development process, FDA may need to provide additional guidance on the extent and type of evidence necessary to validate that products are working as intended.72
To fully seize the potential benefits that AI can add to the health care field while simultaneously ensuring the safety of patients, FDA may need to forge partnerships with a variety of stakeholders, including hospital accreditors, private technology firms, and other government actors such as the Office of the National Coordinator for Health Information Technology, which promulgates key standards for many software products, or the Centers for Medicare and Medicaid Services, which makes determinations about which technologies those insurance programs will cover. And, as previously mentioned, Congress may need to grant FDA additional authorities before the agency can implement some of its proposed policies, particularly as they relate to the precertification pilot.
Conclusion
AI represents a transformational opportunity to improve patient outcomes, drive efficiency, and expedite research across health care. As such, health care providers, software developers, and researchers will continue to innovate and develop new AI products that test the current regulatory framework. FDA is attempting to meet these challenges and develop policies that can enable innovation while protecting public health, but there are many questions that the agency will need to address in order to ensure that this happens. As these policies evolve, legislative action may also be necessary to resolve the regulatory uncertainties within the sector.
Glossary
Explainability: The ability fordevelopers to explain in plain language how their data will be used.73
Generalizability: The accuracy with which results or findings can be transferred to other situations or people outside of those originally studied.74
Good Machine Learning Practices (GMLP): AI/ML best practices (such as those for data management or evaluation), analogous to good software engineering practices or quality system practices.75
Machine learning (ML): An AI technique that can be used to design and train software algorithms to learn from and act on data. These algorithms can be “locked,” so that their function does not change, or “adaptive,” meaning that their behavior can change over time.76
Software as a Medical Device (SaMD): Defined by the International Medical Device Regulators Forum as “software intended to be used for one or more medical purposes that perform these purposes without being part of a hardware medical device.”77
Endnotes
- E.J. Topol, “High-Performance Medicine: The Convergence of Human and Artificial Intelligence,” Nature Medicine 25 (2019): 44–56, https://www.nature.com/articles/s41591-018-0300-7.
- U.S. Food and Drug Administration, “Artificial Intelligence/Machine Learning (AI/ML)-Based Software as a Medical Device (SaMD) Action Plan” (2021), https://www.fda.gov/media/145022/download; U.S. Food and Drug Administration, “Proposed Regulatory Framework for Modifications to Artificial Intelligence/Machine Learning (AI/ML)-Based Software as a Medical Device (SaMD)—Discussion Paper and Request for Feedback” (2019), https://www.fda.gov/media/122535/download.
- G. Daniel et al., “Current State and Near-Term Priorities for AI-Enabled Diagnostic Support Software in Health Care” (Duke-Margolis Center for Health Policy, 2019), https://healthpolicy.duke.edu/sites/default/files/2019-11/dukemargolisaienableddxss.pdf.
- K.-H. Yu, A.L. Beam, and I.S. Kohane, “Artificial Intelligence in Healthcare,” Nature Biomedical Engineering 2 (2018): 719–31, https://www.nature.com/articles/s41551-018-0305-z.
- L. Malone, “Duke’s Augmented Intelligence System Helps Prevent Sepsis in the ED,” Duke Health, March 10, 2020, https://physicians.dukehealth.org/articles/dukes-augmented-intelligence-system-helps-prevent-sepsis-ed.
- M. Garrity, “U of Maryland Medical Systems Develops Machine Learning Model to Better Predict Readmissions,” June 7, 2019, https://www.beckershospitalreview.com/artificial-intelligence/u-of-maryland-medical-systems-develops-machine-learning-model-to-better-predict-readmissions.html.
- HealthIT.gov, “About ONC’s Cures Act Final Rule,” accessed April 5, 2021, https://www.healthit.gov/curesrule/overview/about-oncs-cures-act-final-rule.
- J. Vamathevan et al., “Applications of Machine Learning in Drug Discovery and Development,” Nature Reviews Drug Discovery 18 (2019): 463–77, https://www.nature.com/articles/s41573-019-0024-5.
- L. Richardson, “Artificial Intelligence Has Helped to Guide Pandemic Response, but Requires Adequate Regulation,” The Pew Charitable Trusts, March 11, 2021, https://www.pewtrusts.org/en/research-and-analysis/articles/2021/03/11/artificial-intelligence-has-helped-to-guide-pandemic-response-but-requires-adequate-regulation; N. Arora, A.K. Banerjee, and M.L. Narasu, “The Role of Artificial Intelligence in Tackling COVID-19,” Future Virology (2020), https://www.ncbi.nlm.nih.gov/pmc/articles/PMC7692869/.
- The first two products listed, COViage and the CLEWICU System, were granted Emergency Use Authorization (EUA) by FDA, which allows developers to market their products during a public health emergency without completing the agency’s standard review process.
- R. Robbins, “FDA Issues Rare Emergency Authorization for an Algorithm Used to Inform COVID-19 Care,” STAT+, Oct. 5, 2020, https://www.statnews.com/2020/10/05/dascena-algorithm-covid19-fda/; RADM Denise M. Hinton, chief scientist, U.S. Food and Drug Administration, letter to Ms. Carol Gu, vice president, Operations, Dascena Inc., “Emergency Use Authorization (EUA) for Emergency Use of the COViage Hemodynamic Instability and Respiratory Decompensation Prediction System (COViage),” Sept. 24, 2020, https://www.fda.gov/media/142454/download; Dascena Inc., “Dascena Receives FDA EUA for COVID-19 Hemodynamic Instability and Respiratory Decompensation Prediction System,” Oct. 2, 2020, https://www.dascena.com/press-releases-old-dev/dascena-receives-fda-eua-for-covid-19-hemodynamic-instability-and-respiratory-decompensation-prediction-system.
- N.P. Taylor, “Emergency Authorization Granted to COVID-19 ICU Prediction Software,” MedTech Dive, May 28, 2020, https://www.medtechdive.com/news/emergency-authorization-granted-to-covid-19-icu-prediction-software/578738/; RADM Denise M. Hinton, chief scientist, U.S. Food and Drug Administration, letter to CLEW Medical Ltd., c/o Ms. Yarmela Pavlovic, Manatt, Phelps & Phillips LLP, “Emergency Use Authorization (EUA) for Emergency Use of the CLEWICU System,” May 26, 2020, https://www.fda.gov/media/138369/download; CLEW, “Clew Receives FDA Emergency Use Authorization (EUA) for Its Predictive Analytics Platform in Support of COVID-19 Patients,” June 16, 2020, https://clewmed.com/clew-receives-fda-emergency-use-authorization-eua-for-its-predictive-analytics-platform-in-support-of-covid-19-patients/.
- Mount Sinai, “Mount Sinai First in U.S. To Use Artificial Intelligence to Analyze Coronavirus (COVID-19) Patients,” May 19, 2020, https://www.mountsinai.org/about/newsroom/2020/mount-sinai-first-in-us-to-use-artificial-intelligence-to-analyze-coronavirus-covid19-patients-pr.
- Epic, “University of Minnesota Develops AI Algorithm to Analyze Chest X-Rays for COVID-19,” Oct. 1, 2020, https://www.epic.com/epic/post/university-minnesota-develops-ai-algorithm-analyze-chest-x-rays-covid-19; University of Minnesota, “University of Minnesota Develops AI Algorithm to Analyze Chest X-Rays for COVID-19,” Oct. 1, 2020, https://twin-cities.umn.edu/news-events/university-minnesota-develops-ai-algorithm-analyze-chest-x-rays-covid-19.
- A. Rajkomar, J. Dean, and I. Kohane, “Machine Learning in Medicine,” The New England Journal of Medicine 380, no. 14 (2019), https://www.nejm.org/doi/full/10.1056/NEJMra1814259.
- Daniel et al., “Current State and Near-Term Priorities for AI-Enabled Diagnostic Support Software in Health Care.”
- Ibid.; Rajkomar, Dean, and Kohane, “Machine Learning in Medicine.”
- Rajkomar, Dean, and Kohane, “Machine Learning in Medicine”; Yu, Beam, and Kohane, “Artificial Intelligence in Healthcare.”
- U.S. Food and Drug Administration, “Artificial Intelligence and Machine Learning in Software as a Medical Device,” last modified Jan. 12, 2021, https://www.fda.gov/medical-devices/software-medical-device-samd/artificial-intelligence-and-machine-learning-software-medical-device.
- U.S. Food and Drug Administration, “Executive Summary for the Patient Engagement Advisory Committee Meeting” (2020), https://www.fda.gov/media/142998/download; M. Matheny et al., “Artificial Intelligence in Health Care: The Hope, the Hype, the Promise, the Peril” (National Academy of Medicine, 2020), https://nam.edu/wp-content/uploads/2019/12/AI-in-Health-Care-PREPUB-FINAL.pdf; Yu, Beam, and Kohane, “Artificial Intelligence in Healthcare.”
- W. Nicholson Price II, “Risks and Remedies for Artificial Intelligence in Health Care,” The Brookings Institution, Nov. 14, 2019, https://www.brookings.edu/research/risks-and-remedies-for-artificial-intelligence-in-health-care/.
- A. Kaushal, R. Altman, and C. Langlotz, “Geographic Distribution of U.S. Cohorts Used to Train Deep Learning Algorithms,” Journal of the American Medical Association 324, no. 12 (2020), https://jamanetwork.com/journals/jama/article-abstract/2770833.
- Nicholson Price II, “Risks and Remedies for Artificial Intelligence in Health Care.”
- D.S. Char, N.H. Shah, and D. Magnus, “Implementing Machine Learning in Health Care—Addressing Ethical Challenges,” New England Journal of Medicine 378, no. 11 (2018): 981–83, https://www.ncbi.nlm.nih.gov/pmc/articles/PMC5962261/.
- A.S. Adamson and A. Smith, “Machine Learning and Health Care Disparities in Dermatology,” JAMA Dermatology 154, no. 11 (2018): 1247-48, https://jamanetwork.com/journals/jamadermatology/article-abstract/2688587.
- W.N.P. II, “Medical AI and Contextual Bias,” University of Michigan Public Law Research Paper No. 632; Harvard Journal of Law & Technology 33, no. 66 (2019), https://papers.ssrn.com/sol3/papers.cfm?abstract_id=3347890#.
- C. Ross, “At Mayo Clinic, Sharing Patient Data With Companies Fuels AI Innovation—and Concerns About Consent,” STAT+, June 3, 2020, https://www.statnews.com/2020/06/03/mayo-clinic-patient-data-fuels-artificial-intelligence-consent-concerns/; S. Gerke, T. Minssen, and G. Cohen, “Ethical and Legal Challenges of Artificial Intelligence-Driven Healthcare,” Artificial Intelligence in Healthcare (2020): 295–336, https://www.ncbi.nlm.nih.gov/pmc/articles/PMC7332220/.
- Ross, “At Mayo Clinic, Sharing Patient Data with Companies Fuels AI Innovation—and Concerns About Consent.”
- U.S. Food and Drug Administration, “Proposed Regulatory Framework for Modifications to Artificial Intelligence/Machine Learning (AI/ML)-Based Software as a Medical Device (SaMD)” (2021), https://www.fda.gov/files/medical devices/published/US-FDA-Artificial-Intelligence-and-Machine-Learning-Discussion-Paper.pdf; Daniel et al., “Current State and Near-Term Priorities for AI-Enabled Diagnostic Support Software in Health Care.”
- Daniel et al., “Current State and Near-Term Priorities for AI-Enabled Diagnostic Support Software in Health Care.”
- Z. Obermeyer et al., “Dissecting Racial Bias in an Algorithm Used to Manage the Health of Populations,” Science 366, no. 6464 (2019): 447-53, https://science.sciencemag.org/content/366/6464/447; C.K. Johnson, “Racial Bias in Health Care Software Aids Whites Over Blacks,” The Seattle Times, Oct. 25, 2019, https://www.seattletimes.com/seattle-news/health/racial-bias-in-health-care-software-aids-whites-over-blacks/.
- S. Samuel, “AI Can Now Outperform Doctors at Detecting Breast Cancer. Here’s Why It Won’t Replace Them,” Vox, Jan. 3, 2020, https://www.vox.com/future-perfect/2020/1/3/21046574/ai-google-breast-cancer-mammogram-deepmind; W.N.P. II, “Regulating Black-Box Medicine,” 116 Michigan Law Review 42 (2017), https://papers.ssrn.com/sol3/papers.cfm?abstract_id=2938391.
- C. Ross, “Could AI Tools for Breast Cancer Worsen Disparities? Patchy Public Data in FDA Filings Fuel Concern,” STAT+, Feb. 11, 2021, https://www.statnews.com/2021/02/11/breast-cancer-disparities-artificial-intelligence-fda/; Centers for Disease Control and Prevention, “Patterns and Trends in Age-Specific Black-White Differences in Breast Cancer Incidence and Mortality—United States, 1999–2014,” Morbidity and Mortality Weekly Report 65, no. 40 (2016): 1093–98, https://www.cdc.gov/mmwr/volumes/65/wr/mm6540a1.htm?CDC_AA_refVal=https%3A%2F%2Fwww.cdc.gov%2Fcancer%2Fdcpc%2Fresearch%2Farticles%2Fbreast_cancer_rates_women.htm.
- R. Robbins and E. Brodwin, “An Invisible Hand: Patients Aren’t Being Told About the AI Systems Advising Their Care,” July 15, 2020, https://www.statnews.com/2020/07/15/artificial-intelligence-patient-consent-hospitals/.
- C. Ross, “Bias, Consent, and Data Transparency: What Patients Want the FDA to Consider About AI in Medicine,” STAT+, Oct. 26, 2020, https://www.statnews.com/2020/10/26/artificial-intelligence-bias-fda-patients/.
- E. Brodwin, “‘It’s Really on Them to Learn’: How the Rapid Rollout of AI Tools Has Fueled Frustration Among Clinicians,” Dec. 17, 2020, https://www.statnews.com/2020/12/17/artificial-intelligence-doctors-nurses-frustrated-ai-hospitals/.
- U.S. Food and Drug Administration, “Proposed Regulatory Framework for Modifications to Artificial Intelligence/Machine Learning (AI/ML)-Based Software as a Medical Device (SaMD).”
- U.S. Food and Drug Administration, “Executive Summary for the Patient Engagement Advisory Committee Meeting.”
- U.S. Food and Drug Administration, “What Are Examples of Software as a Medical Device?” last modified Dec. 6, 2017, https://www.fda.gov/medical-devices/software-medical-device-samd/what-are-examples-software-medical-device; Deloitte, “Software as a Medical Device,” accessed April 5, 2021, https://www2.deloitte.com/us/en/pages/public-sector/articles/software-as-a-medical-device-fda.html.
- T. Minssen et al., “Regulatory Responses to Medical Machine Learning,” Journal of Law and the Biosciences (2020), https://academic.oup.com/jlb/advance-article/doi/10.1093/jlb/lsaa002/5817484.
- U.S. Food and Drug Administration, “Software as a Medical Device (SaMD),” last modified Dec. 4, 2018, https://www.fda.gov/medical-devices/digital-health-center-excellence/software-medical-device-samd; Deloitte, “Software as a Medical Device.”
- U.S. Food and Drug Administration, “FDA Permits Marketing of Artificial Intelligence-Based Device to Detect Certain Diabetes-Related Eye Problems,” April 11, 2018, https://www.fda.gov/news-events/press-announcements/fda-permits-marketing-artificial-intelligence-based-device-detect-certain-diabetes-related-eye; Digital Diagnostics, “IDx-DR,” accessed April 5, 2021, https://dxs.ai/products/idx-dr/idx-dr-overview/.
- U.S. Food and Drug Administration, “FDA Permits Marketing of Artificial Intelligence Algorithm for Aiding Providers in Detecting Wrist Fractures,” May 24, 2018, https://www.fda.gov/news-events/press-announcements/fda-permits-marketing-artificial-intelligence-algorithm-aiding-providers-detecting-wrist-fractures; U.S. Food and Drug Administration, “Evaluation of Automatic Class III Designation for Osteodetect” (https://www.accessdata.fda.gov/cdrh_docs/reviews/DEN180005.pdf.
- U.S. Food and Drug Administration, “FDA Permits Marketing of Clinical Decision Support Software for Alerting Providers of a Potential Stroke in Patients,” Feb. 13, 2018, https://www.fda.gov/news-events/press-announcements/fda-permits-marketing-clinical-decision-support-software-alerting-providers-potential-stroke; U.S. Food and Drug Administration, “Evaluation of Automatic Class III Designation for Contact,” https://www.accessdata.fda.gov/cdrh_docs/reviews/DEN170073.pdf.
- U.S. Food and Drug Administration, “Guardian Connect System—P160007,” last modified April 8, 2018, https://www.fda.gov/medical-devices/recently-approved-devices/guardian-connect-system-p160007; The Medical Futurist, “Medtronic’s Smart Continuous Glucose Monitoring System Rolls Out This Summer,” March 21, 2018, https://medicalfuturist.com/medtronics-smart-continuous-glucose-monitoring-system-rolls-summer/; Medtronic, “The Guardian™ Connect System,” accessed April 5, 2021, https://www.medtronicdiabetes.com/products/guardian-connect-continuous-glucose-monitoring-system.
- Empatica, “Indications for Use and Safety Information,” accessed April 5, 2021, https://www.empatica.com/embrace-IFU; Empatica, “How Does Embrace2 Work? Watch Our Animated Video!” June 7, 2019, https://www.empatica.com/blog/how-does-embrace2-work-watch-our-animated-video.html
- I.V. Loon, “FibriCheck Receives FDA Clearance for Its Digital Heart Rhythm Monitor,” FibriCheck, Oct. 8, 2018, https://www.fibricheck.com/fibricheck-receives-fda-clearance-for-its-digital-heart-rhythm-monitor/.
- Daniel et al., “Current State and Near-Term Priorities for AI-Enabled Diagnostic Support Software in Health Care”; for example: T. Mills, director, Division of Radiological Health, Office of In Vitro Diagnostics and Radiological Health, Center for Devices and Radiological Health, letter to Kevin Harris, CEO, CureMetrix Inc., “Reply to Section 510(k) Premarket Notification of Intent,” March 8, 2019, http://www.accessdata.fda.gov/cdrh_docs/pdf18/K183285.pdf.
- U.S. Food and Drug Administration, “Overview of Device Regulation,” last modified Sept. 4, 2020, https://www.fda.gov/medical-devices/device-advice-comprehensive-regulatory-assistance/overview-device-regulation; U.S. Food and Drug Administration, “Step 3: Pathway to Approval,” last modified Feb. 9, 2018, https://www.fda.gov/patients/device-development-process/step-3-pathway-approval.
- S. Benjamens, P. Dhunnoo, and B. Meskó, “The State of Artificial Intelligence-Based FDA-Approved Medical Devices and Algorithms: An Online Database,” npj Digital Medicine, Vol.3, no. 118 (2018), https://www.nature.com/articles/s41746-020-00324-0.
- U.S. Food and Drug Administration, “Step 3: Pathway to Approval”; Daniel et al., “Current State and Near-Term Priorities for AI-Enabled Diagnostic Support Software in Health Care.”
- U.S. Food and Drug Administration, “De Novo Classification Request for IDx-DR” (2018), https://www.accessdata.fda.gov/cdrh_docs/reviews/DEN180001.pdf; U.S. Food and Drug Administration, “Evaluation of Automatic Class III Designation for Osteodetect”; U.S. Food and Drug Administration, “Evaluation of Automatic Class III Designation for Contact.”
- J. Jin, “FDA Authorization of Medical Devices,” JAMA 311, no. 4 (2014): 435, https://jamanetwork.com/journals/jama/fullarticle/1817798; U.S. Food and Drug Administration, “Step 3: Pathway to Approval.”
- C.H. Lias, director, Division of Chemistry and Toxicology Devices, Office of In Vitro Diagnostics and Radiological Health, Center for Devices and Radiological Health, letter to Liane Miller, Medtronic MiniMed, Regulatory Affairs Manager, “Premarket Approval Application (PMA) Review,” https://www.accessdata.fda.gov/cdrh_docs/pdf16/P160007a.pdf.
- Section 520(o) of the Federal Food, Drug, and Cosmetic Act; United States Public Law 114-255—21st Century Cures Act (2016), https://www.congress.gov/114/plaws/publ255/PLAW-114publ255.pdf; U.S. Food and Drug Administration, “Changes to Existing Medical Software Policies Resulting from Section 3060 of the 21st Century Cures Act—Guidance for Industry and Food and Drug Administration Staff” (Sept. 27, 2019), https://www.fda.gov/media/109622/download.
- U.S. Food and Drug Administration, “Clinical Decision Support Software—Draft Guidance for Industry and Food and Drug Administration Staff” (2019), https://www.fda.gov/media/109618/download.
- For example: J.M. Sperl-Hillen et al., “Clinical Decision Support Directed to Primary Care Patients and Providers Reduces Cardiovascular Risk: A Randomized Trial,” Journal of the American Medical Informatics Association 25, no. 9 (2018): 1137-46, https://pubmed.ncbi.nlm.nih.gov/29982627/.
- D. Lim, “Industry Blasts FDA Clinical Decision Software Draft,” Healthcare Dive, Feb. 7, 2018, https://www.healthcaredive.com/news/industry-blasts-fda-clinical-decision-software-draft/516523/; D. Lim, “Latest FDA Clinical Decision Support Software Draft a Step Forward, Industry Says,” Medtech Dive, Jan. 7, 2020, https://www.medtechdive.com/news/latest-fda-clinical-decision-support-software-draft-a-step-forward-industr/569927/.
- U.S. Food and Drug Administration, 21 CFR 807.65(d) (2020), https://www.accessdata.fda.gov/scripts/cdrh/cfdocs/cfcfr/cfrsearch.cfm?fr=807.65.
- Nicholson Price II, “Risks and Remedies for Artificial Intelligence in Health Care.”
- A. Smith, “Using Artificial Intelligence and Algorithms,” Federal Trade Commission, accessed April 8, 2020, https://www.ftc.gov/news-events/blogs/business-blog/2020/04/using-artificial-intelligence-algorithms.
- E. Jillson, “Aiming for Truth, Fairness, and Equity in Your Company’s Use of AI,” Federal Trade Commission, April 19, 2021, https://www.ftc.gov/news-events/blogs/business-blog/2021/04/aiming-truth-fairness-equity-your-companys-use-ai.
- U.S. Food and Drug Administration, “Statement from FDA Commissioner Scott Gottlieb, M.D., and Center for Devices and Radiological Health Director Jeff Shuren, M.D., J.D., on Agency Efforts to Work with Tech Industry to Spur Innovation in Digital Health,” Sept. 12, 2018, https://www.fda.gov/news-events/press-announcements/statement-fda-commissioner-scott-gottlieb-md-and-center-devices-and-radiological-health-director; U.S. Food and Drug Administration, “Statement from FDA Commissioner Scott Gottlieb, M.D., On Steps Toward a New, Tailored Review Framework for Artificial Intelligence-Based Medical Devices,” April 02, 2019, https://www.fda.gov/news-events/press-announcements/statement-fda-commissioner-scott-gottlieb-md-steps-toward-new-tailored-review-framework-artificial; U.S. Food and Drug Administration, “FDA Launches the Digital Health Center of Excellence,” Sept. 22, 2020, https://www.fda.gov/news-events/press-announcements/fda-launches-digital-health-center-excellence.
- U.S. Food and Drug Administration, “Digital Health Software Precertification (Pre-Cert) Program,” Sept. 14, 2020, https://www.fda.gov/medical-devices/digital-health-center-excellence/digital-health-software-precertification-pre-cert-program.
- Senator Elizabeth Warren, Senator Patty Murray, and Senator Tina Smith, letter to Scott Gottlieb, commissioner, U.S. Food and Drug Administration, and Jeffrey Shuren, director, Center for Devices and Radiological Health, U.S. Food and Drug Administration, “Letter to FDA on Regulation of Software as Medical Device,” Oct. 10, 2018, https://www.warren.senate.gov/imo/media/doc/2018.10.10%20Letter%20to%20FDA%20on%20regulation%20of%20sofware%20as%20medical%20device.pdf.
- U.S. Food and Drug Administration, “Artificial Intelligence and Machine Learning in Software as a Medical Device”; U.S. Food and Drug Administration, “Proposed Regulatory Framework for Modifications to Artificial Intelligence/Machine Learning (AI/ML)-Based Software as a Medical Device (SaMD).”
- U.S. Food and Drug Administration, 21 CFR 820 (2020), https://www.accessdata.fda.gov/scripts/cdrh/cfdocs/cfcfr/CFRSearch.cfm?CFRPart=820&showFR=1&subpartNode=21:8.0.1.1.12.1.
- G. Slabodkin, “FDA AI-Machine Learning Strategy Remains Work in Progress,” Medtech Dive, accessed Sept. 14, 2020, https://www.medtechdive.com/news/fda-ai-machine-learning-strategy-remains-work-in-progress/585146/.
- U.S. Food and Drug Administration, “Proposed Regulatory Framework for Modifications to Artificial Intelligence/Machine Learning (AI/ML)-Based Software as a Medical Device (SaMD)—Discussion Paper and Request for Feedback”; U.S. Food and Drug Administration, “Developing the Software Precertification Program: Summary of Learnings and Ongoing Activities” (2020), https://www.fda.gov/media/142107/download.
- Slabodkin, “FDA AI-Machine Learning Strategy Remains Work in Progress.”
- U.S. Food and Drug Administration, “FDA Releases Artificial Intelligence/Machine Learning Action Plan,” Jan. 12, 2021, https://www.fda.gov/news-events/press-announcements/fda-releases-artificial-intelligencemachine-learning-action-plan; U.S. Food and Drug Administration, “Artificial Intelligence/Machine Learning (AI/ML)-Based Software as a Medical Device (SaMD) Action Plan.”
- U.S. Government Accountability Office and National Academy of Medicine, “Artificial Intelligence in Health Care Benefits and Challenges of Machine Learning in Drug Development” (2019),www.gao.gov/assets/gao-20-215sp.pdf.
- U.S. Food and Drug Administration, “Executive Summary for the Patient Engagement Advisory Committee Meeting.”
- Ibid.
- U.S. Food and Drug Administration, “Proposed Regulatory Framework for Modifications to Artificial Intelligence/Machine Learning (AI/ML)-Based Software as a Medical Device (SaMD) —Discussion Paper and Request for Feedback.”
- U.S. Food and Drug Administration, “Artificial Intelligence and Machine Learning in Software as a Medical Device.”
- International Medical Device Regulators Forum, “Software as a Medical Device (SaMD): Key Definitions” (2013), http://www.imdrf.org/docs/imdrf/final/technical/imdrf-tech-131209-samd-key-definitions-140901.pdf; U.S. Food and Drug Administration, “Software as a Medical Device (SaMD).”